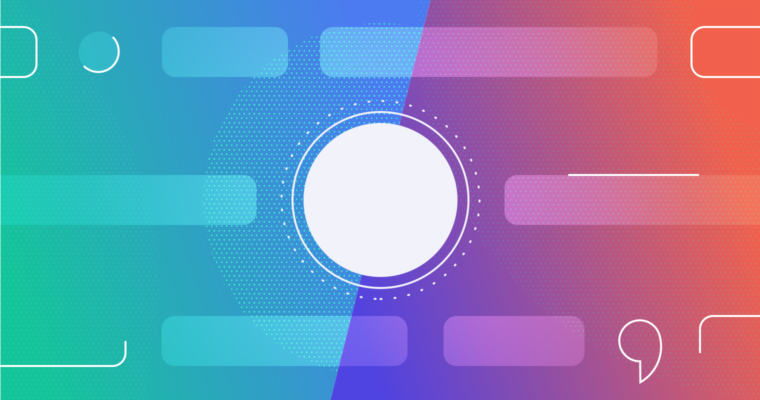
At some point in your life, you may have had a teacher who railed against a particular error in English writing: run-on sentences.
Run-ons are a common type of error. Among college students in the United States, run-on sentences are the eighteenth most frequent error made by native English speakers and the eighth-most frequent error made by students who are not native English speakers.
The ability to automatically detect and fix this type of error would obviously be useful to writers. But there are even broader applications. When you dictate a text message, for example, you need to say “period” at the end of your sentence before starting a new one, or else your transcription turns into one long run-on. An AI system that can automatically figure out where a sentence should start and stop could automatically insert the proper punctuation, freeing up your brain to concentrate on the information you’re trying to communicate.
Grammarly’s work on run-on sentences is the subject of a new paper we presented at The 4th Workshop on Noisy User-generated Text last week at the EMNLP conference in Brussels. We’re proud to say that it won one of the two best paper awards at the workshop! Read on to see how Grammarly is tackling the challenge of correcting run-on sentences.
What is a run-on sentence?
The definition of a run-on sentence varies a bit from person to person. Some people consider comma splices a type of run-on sentence. To others, a run-on sentence is simply a very long sentence. Length alone, however, does not make a sentence a true run-on.
Essentially, a run-on sentence is just two or more complete sentences that have been improperly squashed together. Here’s an example of a run-on:
There are two independent clauses here: Live life to the fullest and don’t take anything for granted. Traditionally, when you want to join two independent clauses together, you need to link them together in some way. One option is to use a comma and a conjunction:
Another option is to use a semicolon:
The third option is to break the clauses into separate sentences:
The problem with run-on sentences is that they’re hard to understand. Conjunctions, semicolons, and periods act as signposts within a sentence to help readers follow what the writer is saying. When these signposts are absent, it’s likely that readers will need to backtrack and reread to make sense of the sentence.
Why it’s hard to automatically correct run-ons
Grammarly already corrects punctuation mistakes and grammatical errors. So what’s different about teaching an AI system to fix run-on sentences? Why is it so hard?
Many punctuation or grammatical errors affect only an isolated part of a sentence. That means your AI system only needs to process a particular chunk of the sentence in order to identify and fix the problem. A run-on, though, is a sentence-level problem. It requires your AI to process a much longer and more complex string of text.
Automatically fixing run-ons is also difficult because there are multiple ways to do it. As in the example above, you can add punctuation, a conjunction, or break the run-on into multiple sentences. Your AI will need to learn how to identify the best way to fix a run-on in a particular situation.
On top of that, there’s just not a lot of existing data out there to train AI systems on for this purpose. Although run-on sentences are common mistakes, there was no existing corpus that included enough labeled run-on sentences to use as training data. (A corpus is a large collection of text that has been labeled in a way that computer algorithms can learn from.)
What we did
The first order of business was to create a collection of run-on sentences. We artificially generated run-on sentences by removing the punctuation between pairs of sentences from a corpus of news articles. (See our paper for a full explanation of our process and how we selected candidate sentences.)
We then used our newly created run-on sentences to train the two machine-learning models we built to identify and correct run-ons. Machine learning is an area of AI that involves teaching an algorithm to perform tasks automatically by showing it lots of examples rather than by providing a series of rigidly predefined steps.
Correcting run-on sentences: What we found
Once the models were trained, we tested them on a new set of artificially created run-on sentences as well as a small set of naturally occurring run-on sentences from an existing research corpus.
We found that both of them outperformed leading models for punctuation restoration and grammatical error correction on this task. There was also another exciting finding: Our models, which were trained on artificially generated sentences, were able to identify run-on sentences written by real writers just as well as they identified artificial run-on sentences.
There is, of course, more work to be done here. Our training data was generated using “clean” text, meaning that the text contained no grammatical errors other than the ones we inserted. In the real world, run-on sentences may contain additional grammatical problems that make it harder for algorithms to identify and fix the run-on. Nevertheless, this is an exciting step toward our vision of creating a comprehensive communication assistant that helps you write messages that will be understood exactly as you intended.
How do you correct run-on sentences it’s not as easy as it seems is a new paper by Junchao Zheng, Courtney Napoles, Joel Tetreault, and Kostiantyn Omelianchuk. It was presented at the Fourth Workshop on Noisy User-generated Text co-located with EMNLP 2018. The paper appears in the Proceedings of the 2018 EMNLP Workshop W-NUT: The Fourth Workshop on Noisy User-generated Text.
More from our Under the Hood at Grammarly series: