Research at Grammarly
Grammarly builds unmatched AI-enabled writing assistance that helps people across platforms and devices, and our large and growing customer base reflects our edge. Grammarly’s support goes far beyond writing mechanics, combining rule-based approaches to explore uncharted areas in advanced machine learning. Our newest features leverage the power of generative AI to solve real customer problems.
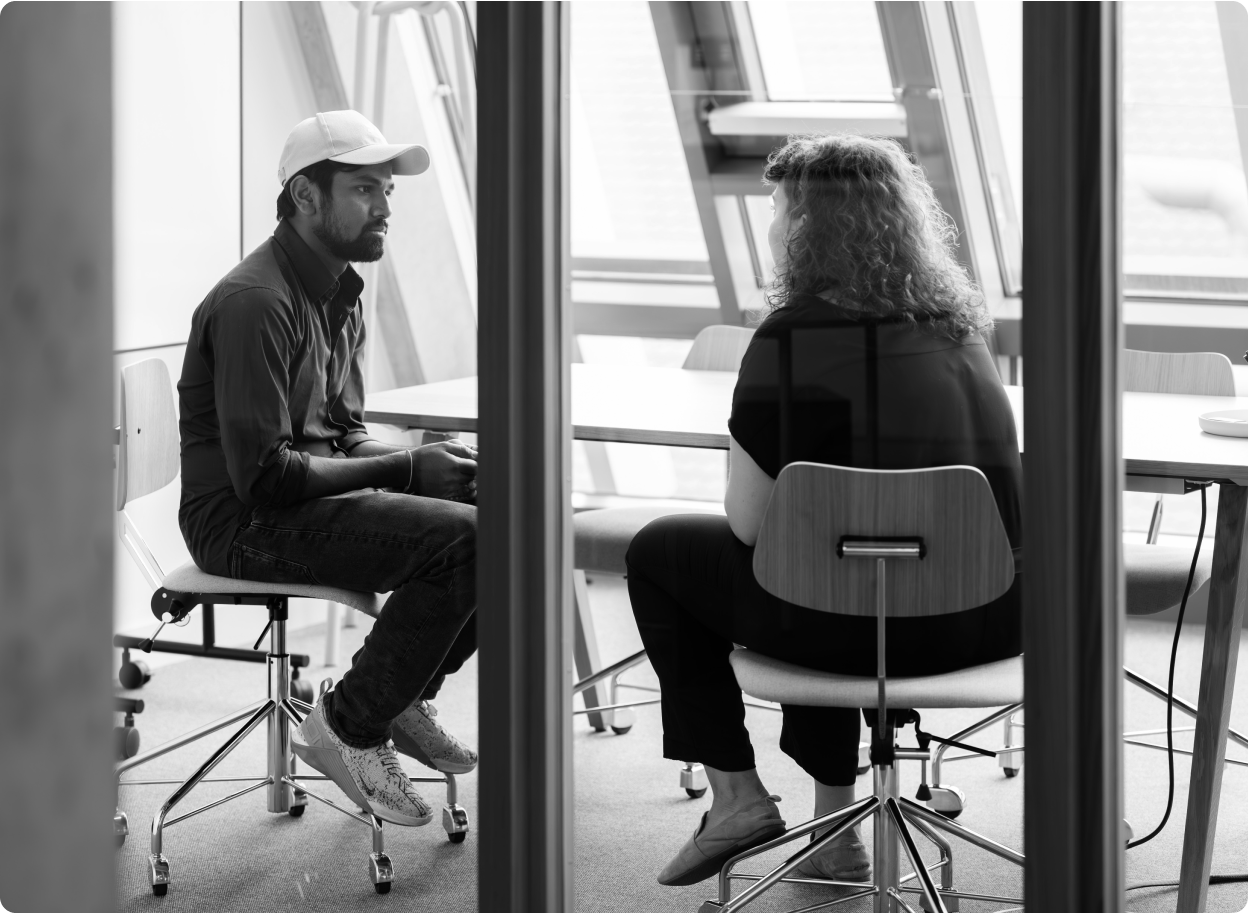
Who We Are
Continuous innovation makes Grammarly a market leader.
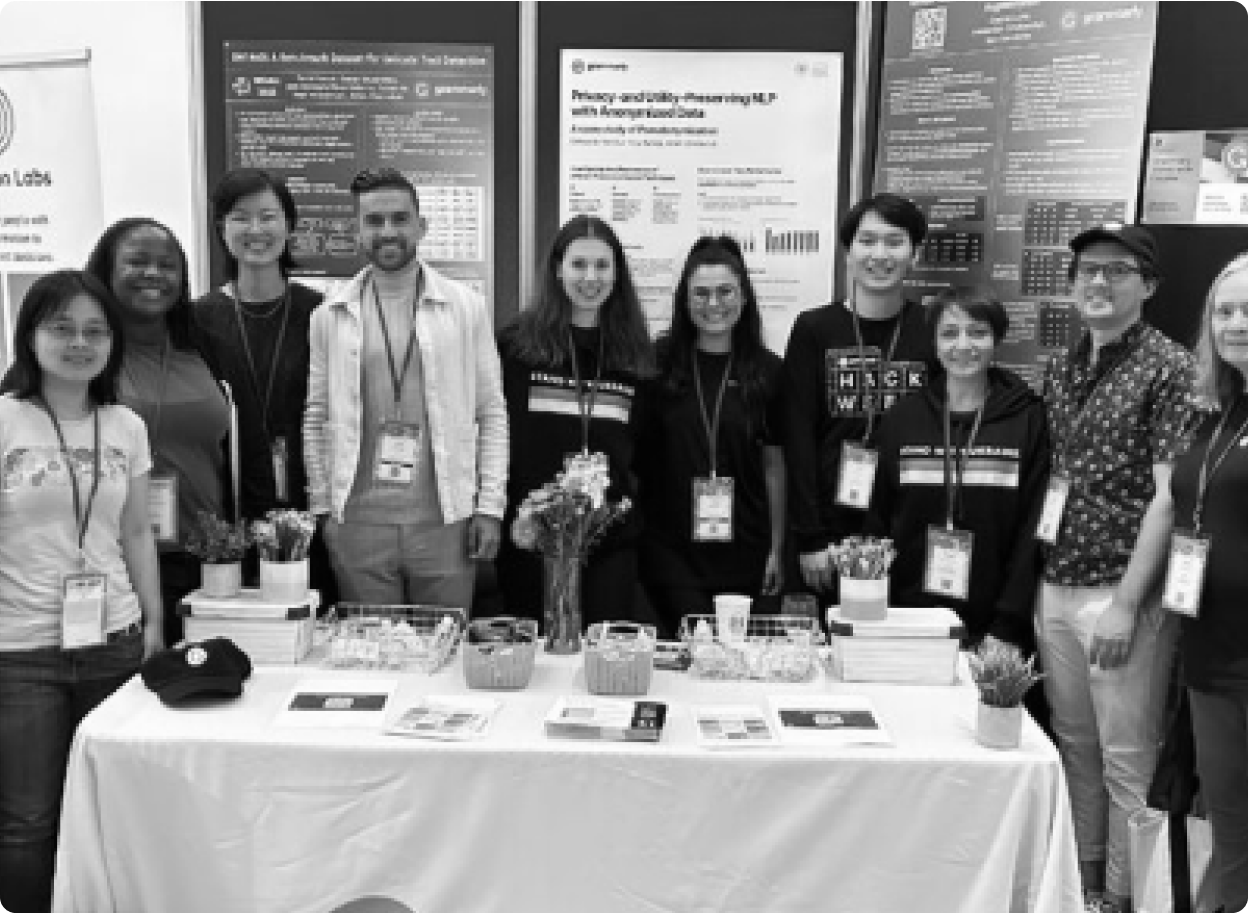
Our team comprises researchers, linguists, and other functional roles. With diverse backgrounds in natural language processing, machine learning, and computational linguistics, together we advance research that revolutionizes the way people digitally communicate and uphold Grammarly’s vision of improving people’s lives through improved communication.
Our Applied Research team has been engaging in research for more than ten years. Our work directly impacts Grammarly’s products, which help millions of people worldwide. We further contribute to society and the research community by publishing, releasing open-source code, and sharing unique models and datasets.
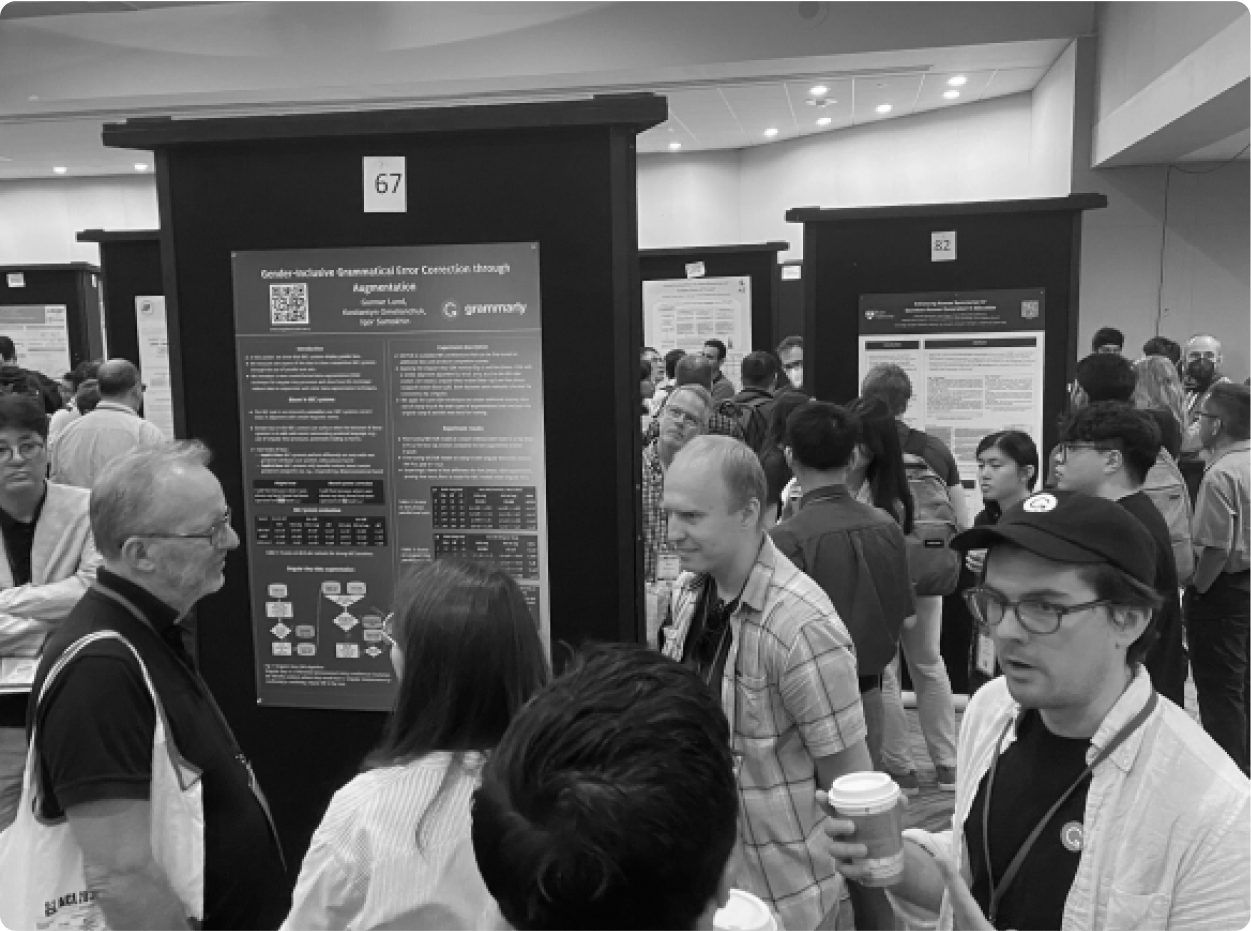
Bring your talent to Grammarly and help us lead the future of AI writing assistance.
Recent Publications
ContraDoc: Understanding Self-Contradictions in Documents With Large Language Models
Authors: Jierui Li, Vipul Raheja, Dhruv Kumar
Authors: Jierui Li, Vipul Raheja, Dhruv Kumar
CoEdIT: Text Editing by Task-Specific Instruction Tuning
Authors: Vipul Raheja, Dhruv Kumar, Ryan Koo, Dongyeop Kang
Conference: EMNLP 2023
Authors: Vipul Raheja, Dhruv Kumar, Ryan Koo, Dongyeop Kang
Conference: EMNLP 2023
Speakerly™: A Voice-Based Writing Assistant for Text Composition
Authors: Dhruv Kumar*, Vipul Raheja*, Alice Kaiser-Schatzlein, Robyn Perry, Apurva Joshi, Justin Hugues-Nuger, Samuel Lou, Navid Chowdhury
Conference: EMNLP 2023
Authors: Dhruv Kumar*, Vipul Raheja*, Alice Kaiser-Schatzlein, Robyn Perry, Apurva Joshi, Justin Hugues-Nuger, Samuel Lou, Navid Chowdhury
Conference: EMNLP 2023
DeTexD: A Benchmark Dataset for Delicate Text Detection
Authors: Serhii Yavnyi, Oleksii Sliusarenko, Jade Razzaghi, Olena Nahorna, Yichen Mo, Knar Hovakimyan, Artem Chernodub
Conference: WOAH @ ACL 2023
Authors: Serhii Yavnyi, Oleksii Sliusarenko, Jade Razzaghi, Olena Nahorna, Yichen Mo, Knar Hovakimyan, Artem Chernodub
Conference: WOAH @ ACL 2023
Gender-Inclusive Grammatical Error Correction Through Augmentation
Authors: Gunnar Lund, Kostiantyn Omelianchuk, Igor Samokhin
Conference: BEA 2023 @ ACL 2023
Authors: Gunnar Lund, Kostiantyn Omelianchuk, Igor Samokhin
Conference: BEA 2023 @ ACL 2023
Privacy-and Utility-Preserving NLP With Anonymized Data: A Case Study of Pseudonymization
Authors: Oleksandr Yermilov, Vipul Raheja, Artem Chernodub
Conference: TrustNLP @ ACL 2023
Authors: Oleksandr Yermilov, Vipul Raheja, Artem Chernodub
Conference: TrustNLP @ ACL 2023
UA-GEC: Grammatical Error Correction and Fluency Corpus for the Ukrainian Language
Authors: Oleksiy Syvokon, Olena Nahorna, Pavlo Kuchmiichuk, Nastasiia Osidach
Conference: UNLP @ EACL 2023
Authors: Oleksiy Syvokon, Olena Nahorna, Pavlo Kuchmiichuk, Nastasiia Osidach
Conference: UNLP @ EACL 2023
Writing Assistants Should Model Social Factors of Language
Authors: Vivek Kulkarni, Vipul Raheja
Conference: In2Writing @ CHI 2023
Authors: Vivek Kulkarni, Vipul Raheja
Conference: In2Writing @ CHI 2023
Improving Iterative Text Revision by Learning Where to Edit From Other Revision Tasks
Authors: Zae Myung Kim, Wanyu Du, Vipul Raheja, Dhruv Kumar, Dongyeop Kang
Conference: EMNLP 2022
Authors: Zae Myung Kim, Wanyu Du, Vipul Raheja, Dhruv Kumar, Dongyeop Kang
Conference: EMNLP 2022
Read, Revise, Repeat: A System Demonstration for Human-in-the-Loop Iterative Text Revision
Authors: Wanyu Du, Zae Myung Kim, Vipul Raheja, Dhruv Kumar, Dongyeop Kang
Conference: In2Writing @ ACL 2022
Authors: Wanyu Du, Zae Myung Kim, Vipul Raheja, Dhruv Kumar, Dongyeop Kang
Conference: In2Writing @ ACL 2022
Understanding Iterative Revision From Human-Written Text
Authors: Wanyu Du, Vipul Raheja, Dhruv Kumar, Zae Myung Kim, Melissa Lopez, Dongyeop Kang
Conference: ACL 2022
Authors: Wanyu Du, Vipul Raheja, Dhruv Kumar, Zae Myung Kim, Melissa Lopez, Dongyeop Kang
Conference: ACL 2022
Ensembling and Knowledge Distilling of Large Sequence Taggers for Grammatical Error Correction
Authors: Maks Tarnavskyi, Artem Chernodub, Kostiantyn Omelianchuk
Conference: ACL 2022
Authors: Maks Tarnavskyi, Artem Chernodub, Kostiantyn Omelianchuk
Conference: ACL 2022
Open Source and Datasets
IteraTeR (2022), R3 (2022), DELIteraTeR (2022): Iterative Text Revision (IteraTeR) From Human-Written Text.
- GitHub repo
- Datasets: Full Sentences, Human Sentences, Full Doc, Human Doc
- Models: Roberta Intention Classifier, BART Revision Generator, PEGASUS Revision Generator, R3 Binary Classifier, R3 Intent Classifier
Pseudonymization (2023): This work investigates the effectiveness of different pseudonymization techniques, ranging from rule-based substitutions to using pretrained large language models on a variety of datasets and models used for two widely used NLP tasks: text classification and summarization.
Grammarly NLP researchers found evidence of bias in off-the-shelf grammatical error correction systems: they performed worse for sentences with the gender-neutral pronoun “They.” So, we developed a technique to create augmented datasets with many examples of the singular “they.” We hope others use it to make NLP systems more gender-inclusive.
GECToR: Grammatical Error Correction: Tag, Not Rewrite. The GitHub repository provides code for training and testing state-of-the-art models for grammatical error correction with the official PyTorch implementation of the paper titled “GECToR: Grammatical Error Correction: Tag, Not Rewrite.”
UA-GEC Corpus (2021): Grammatical Error Correction and Fluency Corpus for the Ukrainian Language
CoEdIT (2023): Text Editing by Task-Specific Instruction Tuning
- GitHub repo: The official repository providing datasets, models, and code for CoEdIT, the instruction-tuned text editing models.
- Models: L, XL, XXL, XL-composite
- Dataset
Internship Program
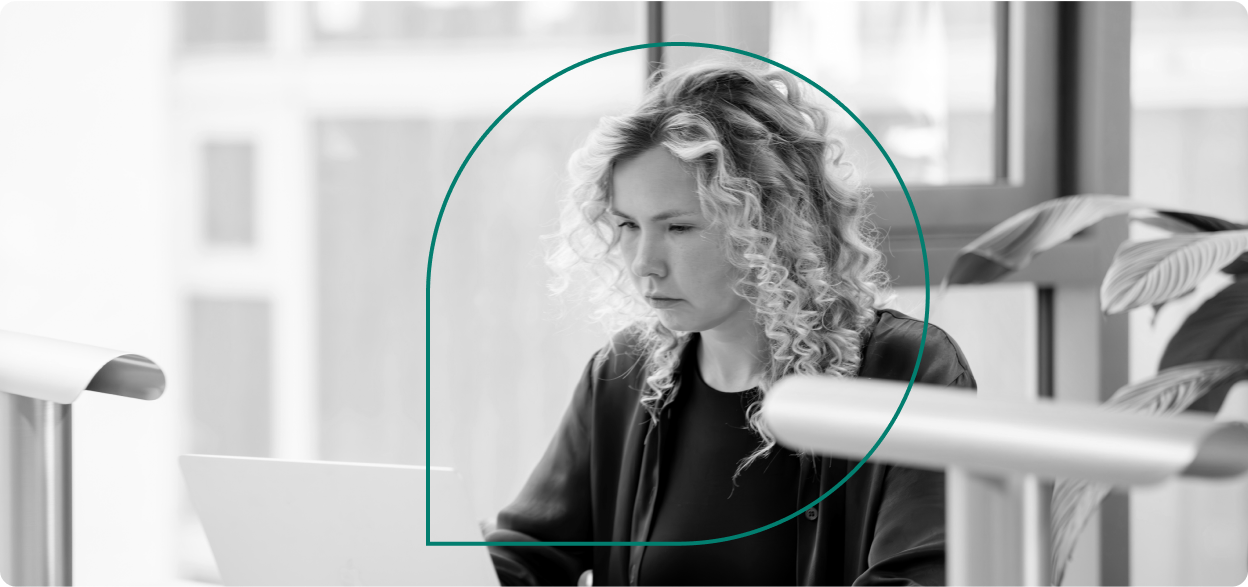
Grammarly has had a successful PhD internship program for many years; see publications above. In 2023, we had five interns pursuing PhDs in machine learning and one in linguistics.
We are starting our 2024 summer internship program soon; please check your eligibility and apply here.
We are starting our 2024 summer internship program soon; please check your eligibility and apply here.
Understand Our Design Process
Our Engineering blog is a great place to gain insight into the creative challenges and solutions that unlock the potential of our product.
And it’s all written by our very own builders and makers, for builders and makers like you.
And it’s all written by our very own builders and makers, for builders and makers like you.
Stay In Touch
Stay connected with Grammarly’s vibrant community—follow us on LinkedIn and sign up for our Engineering Digest for news and updates on online meetups, offline events, and more. We believe in the power of networking and look forward to sharing our journey with you!