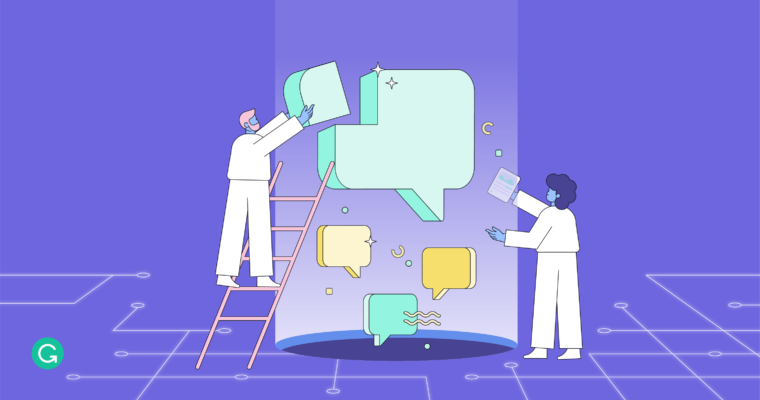
This article was co-written by Yury Markovsky, Engineering Manager; Timo Mertens, Head of ML and NLP Products; and Chad Mills, Manager, Applied Research and Engineering.
At Grammarly, we are passionate about improving human communication. Core to this mission has been our work in natural language processing (NLP). We rely on our team’s deep expertise in NLP, machine learning (ML), and linguistics to create a delightful product for Grammarly’s 30 million daily active users. But, we feel like we’re only at the beginning. And not just because our models aren’t perfect . . .
A case of curious contractions: “Book” was being modified by a singular (“every”) and a plural (“many”) determiner, triggering a bug (since fixed) in parsing the sentence that affected how our product treated the “n’t.”
. . . but because written communication is an astonishingly complex, multifaceted exchange. Language contains layers of meaning, intertwined with emotion. Both writers and readers can bring different assumptions, contexts, and cultural backgrounds to a text, leaving room for misinterpretation. This is why it’s very possible to write something that doesn’t quite land—that’s syntactically and grammatically correct, but nevertheless fails to communicate the intended message. Adding to these writing challenges is that now, more than ever, communication happens asynchronously and without the benefit of body language—making elements like sarcasm and tone all the more difficult to decipher.
We’re approaching these timely, complex problems head-on. The individuals on our NLP and ML team are essential here. If you’re interested in tackling these obstacles and improving human communication at a massive scale, we’re seeking talented, passionate folks to join Grammarly and boost our NLP and ML efforts. It’s an excellent time to join: We’ve spent over a decade investing in a mature ecosystem of linguistic and machine learning tools, but, because we’re a growing company, individuals still have a lot of ownership. For example, just a handful of people built our tone detector feature, which helps users steer their messages to sound exactly how they intend. Other work that our ML/NLP team developed includes industry-leading grammatical error correction, clarity-improving sentence and paragraph rewrites, and identifying where readers are likely to focus in a text. To make this possible, we’ve built a sophisticated portfolio of production models and a robust system for acquiring, curating, and annotating large amounts of diverse data. And, we’ve cultivated an expert team where each member makes a direct impact. Now we’re applying our mature infrastructure and domain knowledge to many exciting new challenges.
The future of NLP at Grammarly
The vision for Grammarly began with spelling and grammar correction. After achieving state-of-the-art results, we’ve stepped up to higher-order aspects of communication. With features like full-sentence rewrites, tone adjustments, and fluency, we’ve gone from syntax to semantics—from mechanics to meaning. Now we’re looking even more broadly at the steps in creating and interpreting meaning. So far, we’ve focused largely on revision. Next, we want to explore how Grammarly can assist across more stages of the communication process. Could we help people express their goals and ideas with language? Could we help them understand the intended meaning behind each other’s words?
Solving these problems would mean identifying brand-new applications and research directions—not just improving existing models (although we care about that, too). Fortunately, we can prioritize zero-to-one projects and go from concept to launch quickly because we’ve spent over a decade developing an ecosystem of linguistic and machine learning tools. We have well-established practices for accessing and curating data, mitigating bias in our features, and protecting privacy and security. We have built and continue to invest in ML infrastructure to leverage the latest developments across techniques like Transformer-based sequence-to-sequence models, neural machine translation, and massive pre-trained language models.
There’s another challenge here: How do we adapt this complex machinery to address the nuances of different communication verticals? After all, human language is extremely context-driven and context-dependent. One might use domain-specific language at work and different tones with family versus friends. Devices can tell us about how people want to communicate (most are probably more casual when texting, for instance), and they also introduce memory and performance constraints on our models. The more we know about the current task, whether it’s jotting down notes or writing the final draft of a report, the more we can tailor suggestions to specific needs.
Achieving this contextual awareness across many stages of communication means translating general knowledge based on big data into experiences that focus on the individual user. This is an exciting area of research and product development with a lot of open questions, but it’s the kind of work we do best. With over ten years of experience building a delightful communication assistant, we don’t just train a big deep neural network language model to solve every problem. We bring a range of classical and state-of-the-art techniques working in concert to elegantly solve complex challenges—while keeping the customer at the center.
NLP roles at Grammarly
Applied research scientists and machine learning engineers work together on small, nimble teams at Grammarly. They collaborate with computational and analytical linguists and data annotators, as well as product managers, designers, and other cross-functional roles. The partnership with linguists and annotators is instrumental; it ensures team access to unique, high-quality, and curated data for training models. Computational and analytical linguists bring their communication expertise to our NLP problems, so we can blend language rules and heuristics with large-scale statistical methods.
Applied research scientists drive our future roadmap, but they also have an immense practical impact. About half of a research scientist’s time is spent embedded on a project team, building features for end users. The other half, they’re prototyping new directions, experimenting on open-ended ideas, and generally doing higher-risk work that we might otherwise overlook. We take pride in our relationship with the academic community, regularly publish our findings, and maintain a presence at all major NLP conferences (including ACL, EMNLP, and NeurIPS).
Machine learning engineers at Grammarly coordinate entire projects—from developing, training, and testing models to integrating front-end and back-end components into customer-facing features. It’s worth noting that platform engineering is also deeply involved, through ongoing development of new tools and infrastructure for ML projects. On any given day, an ML engineer might be working with research scientists and linguists to explore data, running experiments based on customer feedback, or addressing model scalability and performance. Besides opportunities to deliver amazing new product features from start to finish, there are plenty of interesting model-engineering problems to solve. For example, we’re taking models that were designed to run on a massive server and making them fit on a mobile phone—with trade-offs informed by what we know about how people communicate on different devices.
Human communication is highly subjective and highly personal, and it’s a tremendous product, design, and engineering challenge. But the communication problems we’re solving are exciting because they’re hard—and they’re real. They require creativity and an entrepreneurial spirit, plus a commitment to earning and keeping our users’ trust. We’ve had a lot of success so far, but what we’ve built is only the beginning. It’s a great time to join, own a major part of our future roadmap, and start making an impact on day one.
If you’re ready to help millions around the world communicate better, check out our job openings here.